Assessing information about climate change on a regional scale is easily enabled through General Circulation Models (GCMs). Prioritizing GCMs based on different aspects will aid purpose and current research assesses the operation of GCMs using different performance assessment parameters for ranking. The functioning of 26 GCMs from Coupled Model Intercomparison Project Phase 5 (CMIP5) and 16 GCMs from Coupled Model Intercomparison Project Phase 6 (CMIP6) were evaluated and ranked based on their ability in predicting temperature and precipitation. Cauvery basin from southern peninsular India known for frequent drought and flood are considered in this study. GCMs were ranked using multicriteria compromise programming and identified CanCM4 and CanESM5 as the most credible set of GCMs. Climate change evaluation at a regional scale involves the downscaling of GCMs using various approaches. In this study statistical downscaling using various renowned machine learning techniques is compared with the proposed extreme gradient boosting decision tree technique. GCMs historical simulation is used for downscaling local climate with help of data from daily observation stations located in the study zone. Intercomparison of model performance shows that mean-variance is around 15% for the proposed Extreme Gradient Boosting Decision Tree (EXGBDT) model which is better than other models under consideration. NSE and R2 values fall in the range of 0.75 to 0.85 for calibration and validation periods respectively. Lower variance observed in a comparison of CLIMDEX indices recommends that EXGBDT model performance is better in representing local climatic conditions. Intercomparison of streamflow simulation and prediction of discharge using various renowned machine learning techniques was performed. Model variance and bias observed from the proposed EXGBDT model were less than 15% which is the least compared to other machine learning techniques considered in this study. Model NSE and R2 values are above 0.7 for both the training and testing phase which demonstrates the effectiveness of model performance.
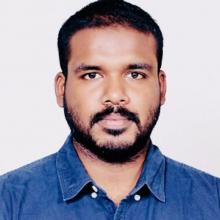
VIT University, Vellore, Tamil Nadu.
Parthiban Loganathan is an active Researcher with five years of experience in research related to Climate change modeling and Environmental sciences. He has completed his Bachelor’s degree in Civil Engineering in 2014, Master’s degree in Energy and Environmental Engineering in 2016 and was awarded Doctor of Philosophy in Environmental and Water Resources Engineering in 2021. His mainstream research is Statistical Downscaling of General Circulation Models, Projection of regional climate for future scenarios, Machine learning based data-driven hydrological models, and Regional Climate Change Assessment. Parthiban has developed expertise in Hydro-climatology, Statistical Modelling, Machine Learning, and R programming language. He has worked with large spatial and temporal datasets related to remote sensing and climate (CMIP5 & CMIP6). He has experience with various statistical machine learning models, graphical data representations, scientific writing, data interpretation, and representation. Parthiban has worked as an Assistant Professor for three years and Research Associate for one year pursuing both academic and research work in the Department of Environmental and Water Resources Engineering, School of Civil Engineering, VIT University, Vellore, Tamil Nadu. Currently, He is working as an Assistant Professor in the Department of Civil Engineering, Sree Vidyanikethan Engineering College, Tirupati, Andhra Pradesh. Parthiban is presently looking for a position in a growing teaching and research group where he can use his skills to their full potential. He always works on identifying the research problems and manages to develop solutions that are effective, economical, and ethical. He has a good ability to work in a research team and can work independently in research investigations. He always prefers to collaborate with fellow researchers to share knowledge, technology, and development.